Privacy in Collaborative ML
Sep 27, 2018
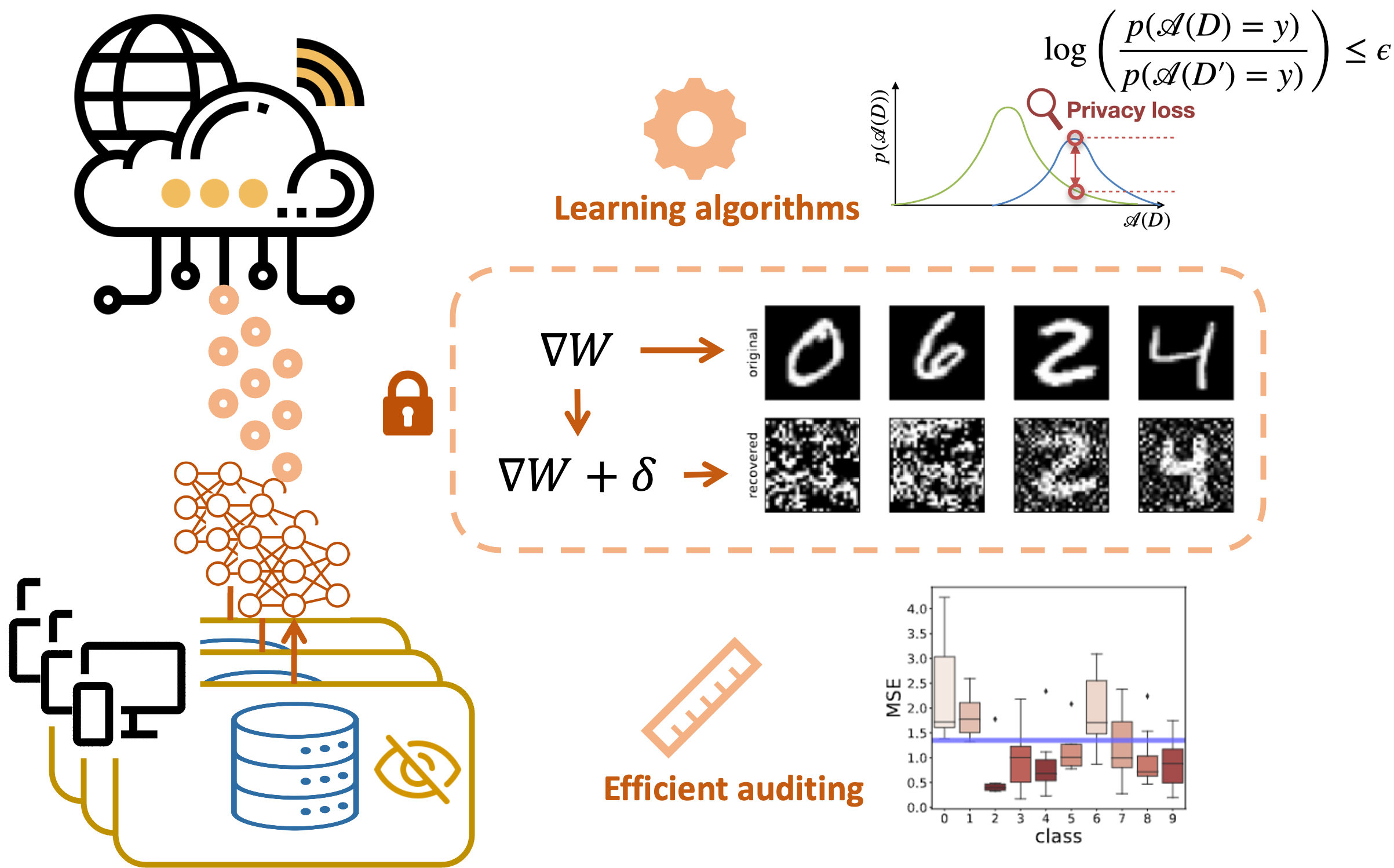
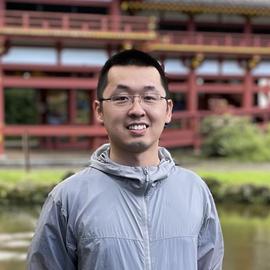
Junyuan "Jason" Hong
Postdoctoral Fellow
My research interest lies in the interaction of human-centered AI and healthcare.
Publications
We propose a new risk to published generative models that finetuning on generated samples can exacerbate the privacy leakage.
Zhangheng Li,
Junyuan "Jason" Hong,
Bo Li,
Zhangyang Wang
We make local LLMs to engineer privacy-preserving prompts that are transferrable for cloud models.
Junyuan "Jason" Hong,
Jiachen T. Wang,
Chenhui Zhang,
Zhangheng Li,
Bo Li,
Zhangyang Wang
We propose a new metric to efficiently evaluate the privacy risks from gradient inversion and provides new insights.
Haobo Zhang,
Junyuan "Jason" Hong,
Yuyang Deng,
Mehrdad Mahdavi,
Jiayu Zhou
We propose a new privacy-preserving learning framework, outsourcing training to cloud without uploading data, which provides more data without injecting noise into gradient or samples.
Junyuan "Jason" Hong,
Lingjuan Lyu,
Jiayu Zhou,
Michael Spranger
Protecting privacy in learning while maintaining the model performance has become increasingly critical in many applications that …
Junyuan "Jason" Hong,
Zhangyang Wang,
Jiayu Zhou
Protecting privacy in gradient-based learning has become increasingly critical as more sensitive information is being used. Many …
Junyuan "Jason" Hong,
Haotao Wang,
Zhangyang Wang,
Jiayu Zhou